Episodes
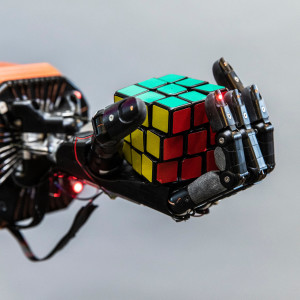
Friday Oct 25, 2019
Friday Oct 25, 2019
Résumé ci-dessous issu de cet épisode de ma newsletter ParlonsFutur
-
Il faut noter que la main robotique elle-même en tant que hardware existe depuis 15 ans, utilisée par de nombreux labos pour leurs expériences
-
l'algorithme lui-même qui permet de savoir quelles faces tourner dans quel sens et dans quel l'ordre, bref l'algo qui permet de résoudre le Rubik's cube, existe depuis 17 ans, un algo codé à la main, et non découvert par apprentissage par la machine, ce n'est pas ce dont il est question ici.
-
De nombreux robots parviennent à résoudre le Rubik's cube, plus vite même que celui d'OpenAI ici (par ex, “a machine developed by MIT solved a cube in less than 0.4 seconds”)
-
Mais aucun robot main humanoïde de ce type y était parvenu (pour un robot avec 24 degrés de liberté dans les mouvement, contre 7 en général pour un bras robot de base), c'est la dextérité du robot main qui est ici révolutionnaire, et la façon dont l'apprentissage s'est fait
-
ici la nouveauté est que le logiciel derrière la main a appris de lui-même comment le faire, via un "apprentissage par renforcement", c'est-à-dire en sachant quel est l'objectif à atteindre, et en se faisant récompenser ou pénaliser plus il se rapproche ou s'éloigne de l'objectif
-
“Human hands let us solve a wide variety of tasks. For the past 60 years of robotics, hard tasks which humans accomplish with their fixed pair of hands have required designing a custom robot for each task. As an alternative, people have spent many decades trying to use general-purpose robotic hardware, but with limited success due to their high degrees of freedom. In particular, the hardware we use here is not new—the robot hand we use has been around for the last 15 years—but the software approach is.”
-
l'autre révolution est que le robot n'a pas eu besoin de tout apprendre dans le monde réel, mais dans une simulation virtuelle, ce qui accélère l'apprentissage puisqu'on peut accélerer le temps dans les simulations et en tenir de très nombreuses en parallèle !
-
OpenAI put it through thousands of years of training in simulation before trying the physical cube solve.
-
But this only takes a few days because we can parallelize the training.
-
You also don’t have to worry about the robots breaking or hurting someone as you’re training these algorithms,”
-
-
"we developed a new method called Automatic Domain Randomization (ADR), which endlessly generates progressively more difficult environments in simulation. This frees us from having an accurate model of the real world, and enables the transfer of neural networks learned in simulation to be applied to the real world”
-
ADR starts with one environment, wherein a neural network learns to solve Rubik’s Cube. As the neural network gets better at the task and reaches a performance threshold, the amount of domain randomization is increased automatically. This makes the task harder, since the neural network must now learn to generalize to more randomized environments.
-
One of the parameters we randomize is the size of the Rubik’s Cube. ADR begins with a fixed size of the Rubik’s Cube and gradually increases the randomization range as training progresses. We apply the same technique to all other parameters, such as the mass of the cube, the friction of the robot fingers, and the visual surface materials of the hand. The neural network thus has to learn to solve the Rubik’s Cube under all of those increasingly more difficult conditions. ADR exposes the network to an endless variety of randomized simulations
-
not only did they change how much gravity there is in the simulation—they changed which way gravity points.
-
We find that our system trained with ADR is surprisingly robust to perturbations even though we never trained with them: The robot can successfully perform most flips and face rotations under all tested perturbations, though not at peak performance.
-
The system can handle situations it never saw during training (comme être embêté par une girafe en peluche, voir vidéo, ou avoir des doigts attachés, etc.) This shows that reinforcement learning isn’t just a tool for virtual tasks, but can solve physical-world problems requiring unprecedented dexterity.
-
Our robot still hasn’t perfected its technique though, as it solves the Rubik’s Cube 60% of the time (and only 20% of the time for a maximally difficult scramble).
-
Un pas vers une IA (et des robots) capable de généraliser ??
-
We believe that meta-learning, or learning to learn, is an important prerequisite for building general-purpose systems, since it enables them to quickly adapt to changing conditions in their environments. The hypothesis behind our methodology ADR is that a memory-augmented networks combined with a sufficiently randomized environment leads to emergent meta-learning, where the network implements a learning algorithm that allows itself to rapidly adapt its behavior to the environment it is deployed in.
-
"We believe that human-level dexterity is on the path towards building general-purpose robots"
-
-
Un pas vers l'explicabilité ? (et la fin du syndrome boîte noire absolue?)
-
Ils ont développé un moyen de visualiser ce qu'il se passe dans la mémoire de l'algo, en temps réel
-
"We find that each memory group has a semantically meaningful behavior associated with it. For example, we can tell by looking at only the dominant group of the network’s memory if it is about to spin the cube or rotate the top clockwise before it happens."
-
Visualizing our networks enables us to understand what they are storing in memory.
-
For example, we can tell by looking at only the dominant group of the network’s memory if it is about to spin the cube or rotate the top clockwise before it happens
-
-
Voir une vidéo du robot résolvant un cube, non éditée, vitesse réelle!
-
Voir la vidéo de présentation des travaux avec toutes les perturbations originales surmontées et non-rencontrées au préalable pendant l'apprentissage
-
Voir l'article sur le site d'OpenAI, avec plein de vidéos sympas
Comments (0)
To leave or reply to comments, please download free Podbean or
No Comments
To leave or reply to comments,
please download free Podbean App.